Dwarf galaxies are small dark matter-dominated galaxies, some of which are embedded within the Milky Way; their lack of baryonic matter (stars and gas) makes them perfect testbeds for dark matter (DM) detection. Understanding the distribution of DM in these systems can be used to pin down microphysical DM interactions that influence the formation and evolution of structures in our Universe. Unfortunately, current observations and current analysis techniques (e.g. Jeans dynamical modeling) fail to pin down the exact DM density profiles in these systems.
For example, an ongoing puzzle in cosmology is the so-called cusp-core problem, referring to whether the inner density profile of DM in dwarf galaxies is a cusp (steeply rising) or a core (flattened). N-body simulations using Lambda-CDM cosmology suggest that in the absence of baryonic (standard) physics, cold DM halos follow the cuspy Navarro-Frenk-White density (NFW), which is characterized by a steep rise in the density. However, recent measurements of stelliar dynamics suggest that these systems could instead have a flattened density profile at their center, also known as a core.
With Prof. Lina Necib and Dr. Siddharth Mishra-Sharma, I develop a new approach for inferring the dark matter density profiles of dwarf galaxies from the obseravable kinematics of stars bound to these systems using graph-based machine learning. Using simulated dwarf galaxies from StarSampler as training data, we contruct a K-Nearest-Neighbor graph for each galaxy, pass it through a Chebyshev Convolutional Neural Network to extract its features, and use these features as conditions for a Normalizng Flow. This allows us to not only predict the dark matter parameters but also their posterior distributions. Below is an example of the posterior distribution predicted by our method:
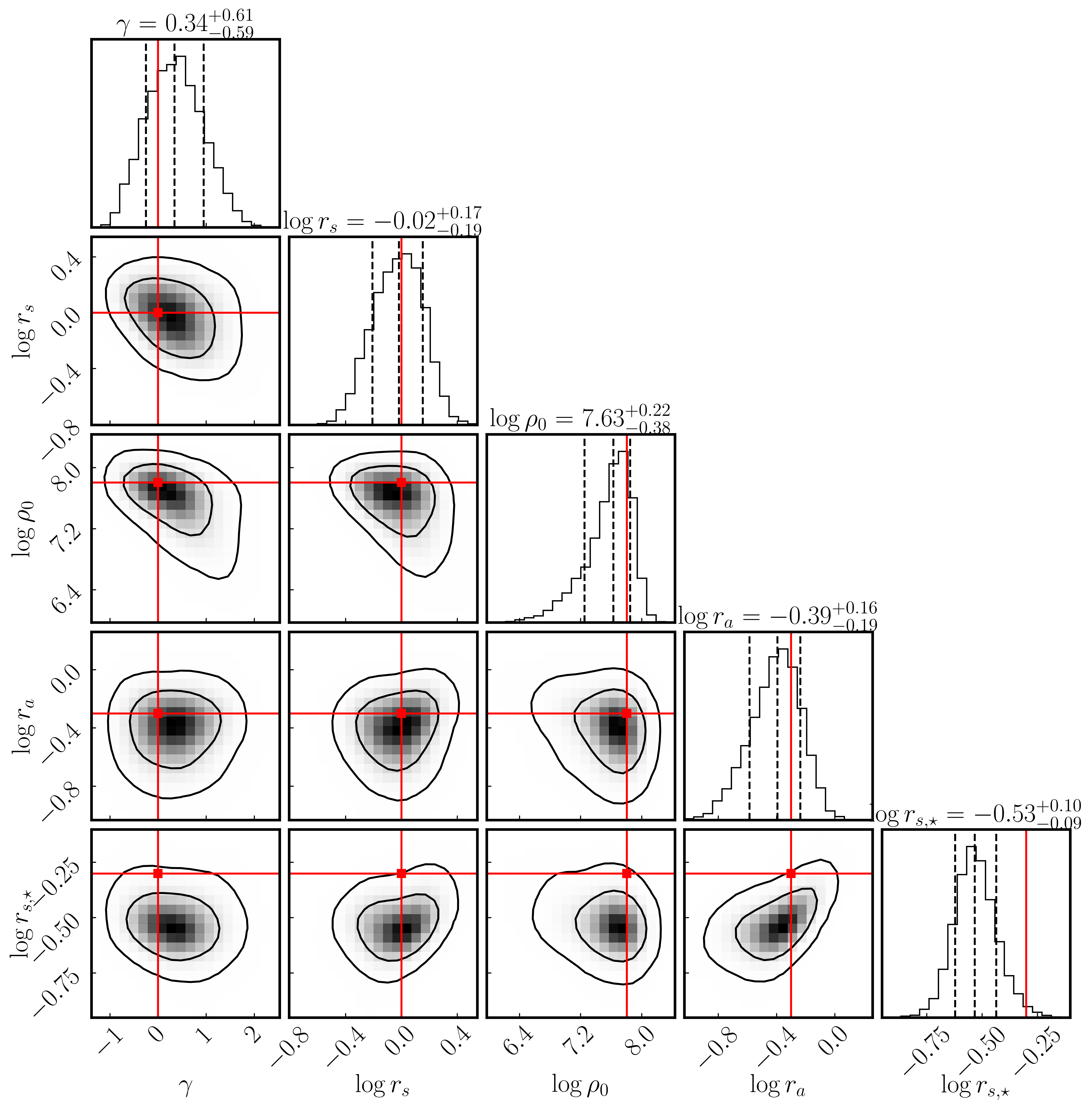
An example posterior distribution of the dark matter parameters and the light profile parameters as predicted by our method.
Our approach aims to address some of the limitations of established methods based on dynamical Jeans modeling such as the necessity of assuming equilibrium and reliance on second-order moments of the stellar velocity distribution. We show that by leveraging more information about the available phase space of bound stars, this method can place stronger constraints on dark matter profiles in dwarf galaxies and has the potential to resolve some of the ongoing puzzles associated with the small-scale structure of dark matter halos.